My AI discriminates? How could this happen and who is to blame? - Marc Hauer (Germany)
Abstract
For some years now, artificial intelligence methods have been used in many areas of daily life. Many applications have been criticized for being discriminatory. There are several ways to deal with such cases: Training datasets can be improved to reduce discriminatory behavior, discriminatory model outputs can be modified post hoc, processes can be established to make discriminatory results usable. In any case, the preliminary assumption is that discrimination can be measured.
The development process of an AI consists of several steps, collection and processing of data is only one of them. Errors can occur at all steps, which have an effect on later steps. This means control processes are needed at all steps and the transitions between them. In addition, responsibilities must be assigned at all these points so that it is clear who must react to errors and problems. At this point, the concept of the „Long Chain of Responsibilities“ is introduced, which helps to clarify these responsibilities.
In this talk, we will talk about how discrimination can enter AI, who is responsible for it, and how discrimination can be operationalized.
Bio Marc Hauer
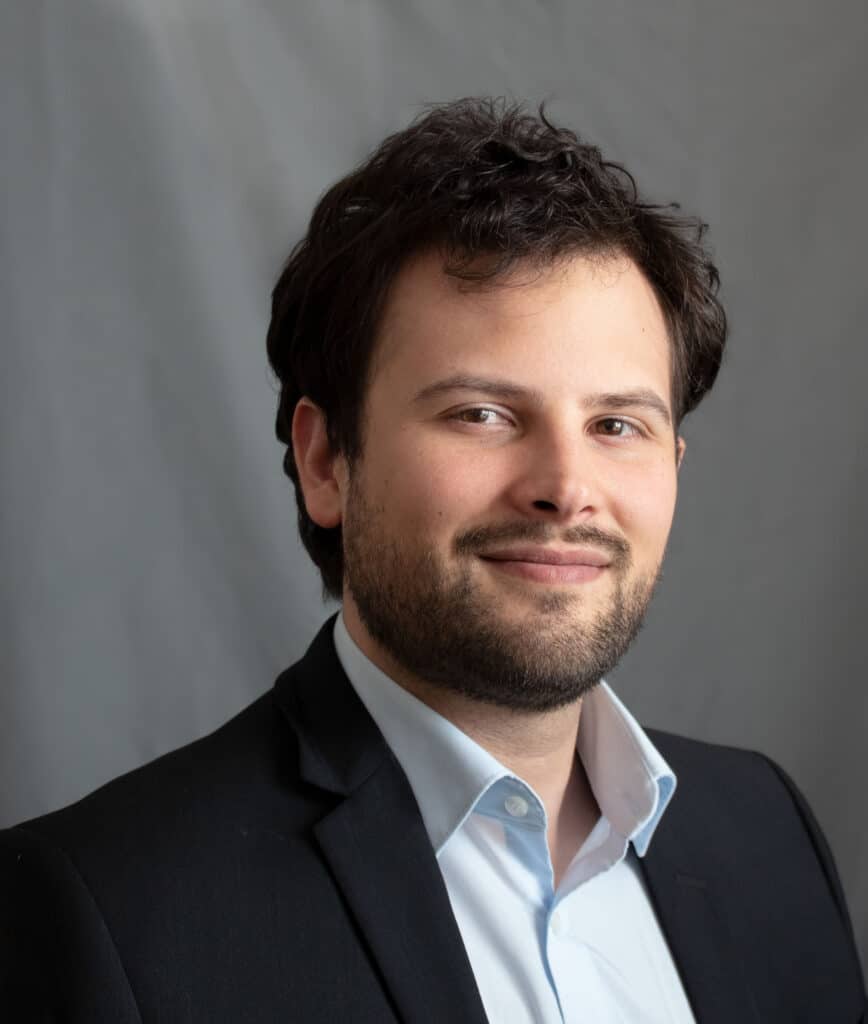
Marc Hauer is a PhD candidate on the question of how to make software development processes and AI products accountable.
Additionally, he works as media education consultant for the Landesmedienzentrum Baden-Württemberg in the education of students, parents and teachers on the topic of computer science and society and for TrustedAI GmbH in AI consulting for companies.
Recording and Slides
A Framework for Exploring the Purposes and Processes of Data Wrangling in Complex Self-Directed Analysis Tasks - Michelle Hoda Wilkerson (USA)
Abstract
The data science education community at large is advocating to include open-ended exploration of large datasets in curriculum and instruction. Introducing this level of flexibility, however, requires teachers and students to wrangle data — that is, to transform complex datasets so that they can be used to particular lines of inquiry.
In this talk, I will draw empirical examples from two data science education projects that engaged teens and young adults in analyzing complex public datasets about socioscientific issues (e.g. public transit, genetics, environmental justice): Data Science Games and Writing Data Stories. Through these examples, I will present a framework for understanding the process of data wrangling as novice analysts determine
(a) whether a particular question can be explored using a given dataset;
(b) what transformation needs to be applied to the dataset in order to pursue that question, and
(c) how to execute that transformation using the tools at hand.
The framework and examples lend insight into how actions by learners that may initially seem inappropriate (such as rejecting a dataset or applying unexpected transformations) can be understood as sensible from the perspective of learners‘ goals and contexts. More generally, they highlight how the interplay of learners‘ investigative goals, the data context, and the available tools all shape the ways in which a complex data investigation unfolds. I will discuss how considering these elements of data exploration in interaction can lead to the more thoughtful development of educational data science tools, activities, and assessment.
Bio Michelle Hoda Wilkerson
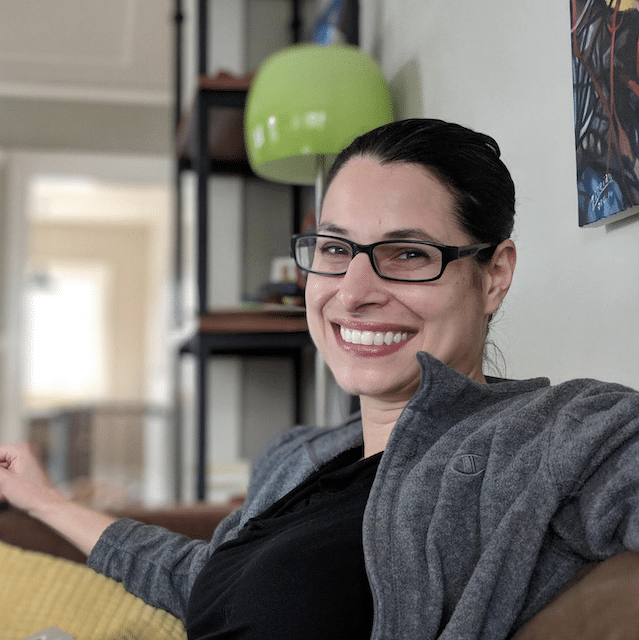
Michelle Hoda Wilkerson is an Associate Professor in the Graduate School of Education and the Graduate Group in Science and Mathematics Education at the University of California, Berkeley. Her research broadly explores the question: How is computing changing what is important to teach and learn in middle and high school science and mathematics classes?
This has led her to study how young people learn with and about scientific computing artifacts such as simulations, data analysis tools, and interactive visualizations. Recently, she has explored how learners‘ relationships with data — for instance as consumers, subjects, and creators of data — shapes how they understand and engage in data analysis. Michelle’s research has been supported by the United States National Science Foundation (NSF), the George Lucas Education Foundation, and Google Education Research. Her work has appeared in general and STEM-specific venues including Educational Researcher, Journal of the Learning Sciences, Science Education, and the Journal of Science Teacher Education and in 2020, her work was recognized with the American Educational Research Association’s Jan Hawkins Award for Humanistic Research and Scholarship in Learning Technologies.