General information on the colloquium
Data science, artificial intelligence, machine learning, data literacy and statistical literacy concerning secondary education are currently discussed in the communities of scientists and educators in statistics, mathematics, computer science, social and natural sciences, and media education. Our colloquium intends to bring together these perspectives and communities to create an interdisciplinary community for scientific exchange.
Since data science and artificial intelligence have become more and more relevant in industrial and economical automation processes, marketing processes, and monitoring in politics, both topics permeate nearly all areas of life. These influences raise questions about future possibilities for social participation, self-determination, and self-realization in the professional and private sector, resulting in the need for educational processes that address these issues in school. For the teaching of mathematics and computer science completely new challenges have emerged, as well as for the subjects of the socio-scientific field and cross-curricular media education.
In our colloquium, we want to take up these issues and discuss state of the art and future trends of education in data science and artificial intelligence that can inspire ideas for teaching data science in secondary schools. We also want to discuss fundamental ideas of data science as they are conceptualized by experts in this field since a broad perspective of data science as a scientific discipline is needed to inform curriculum development. Contributions to the colloquium will also present practice-oriented research as well as research on teachers’ professional development.
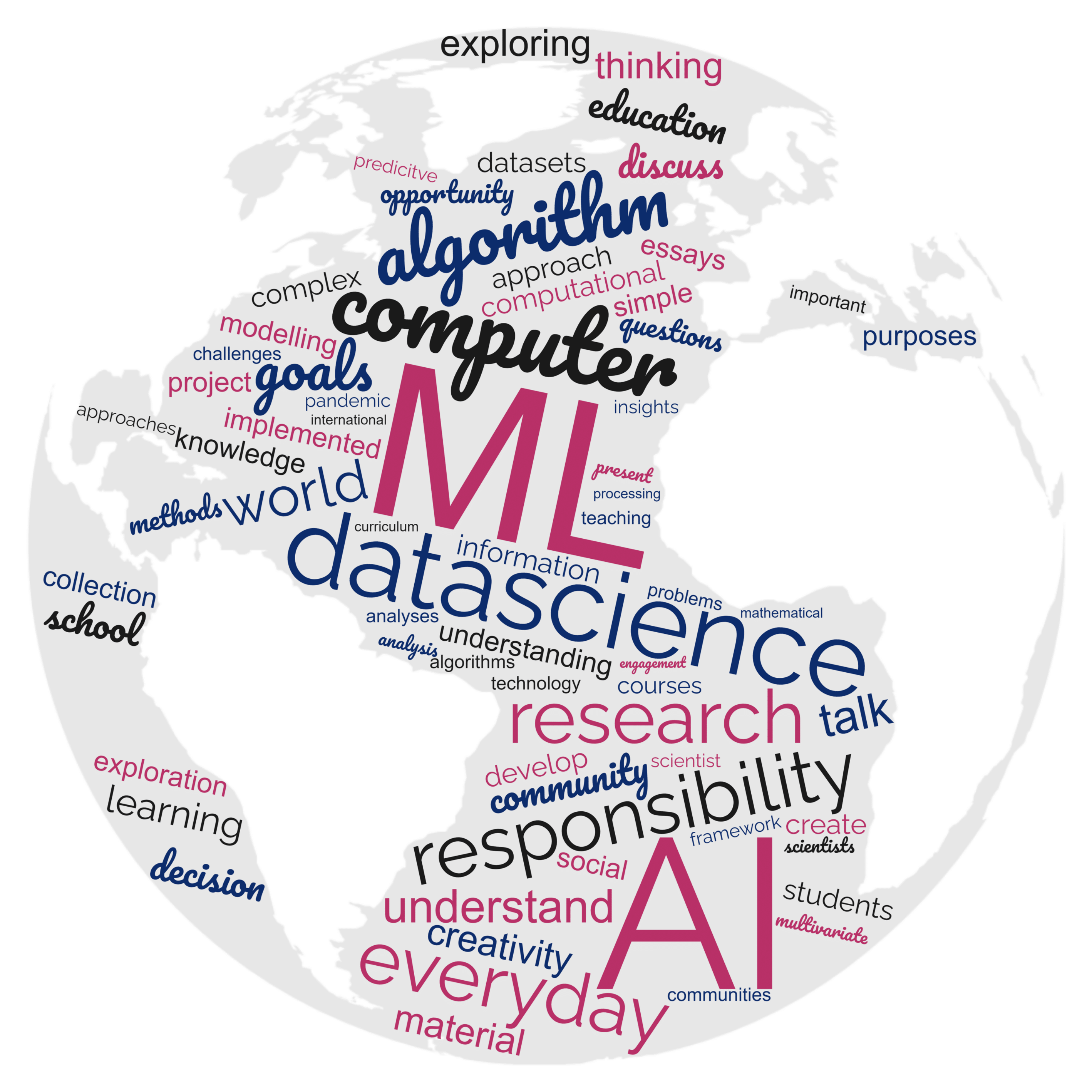